Tip: How to select a future weather file for building performance simulations
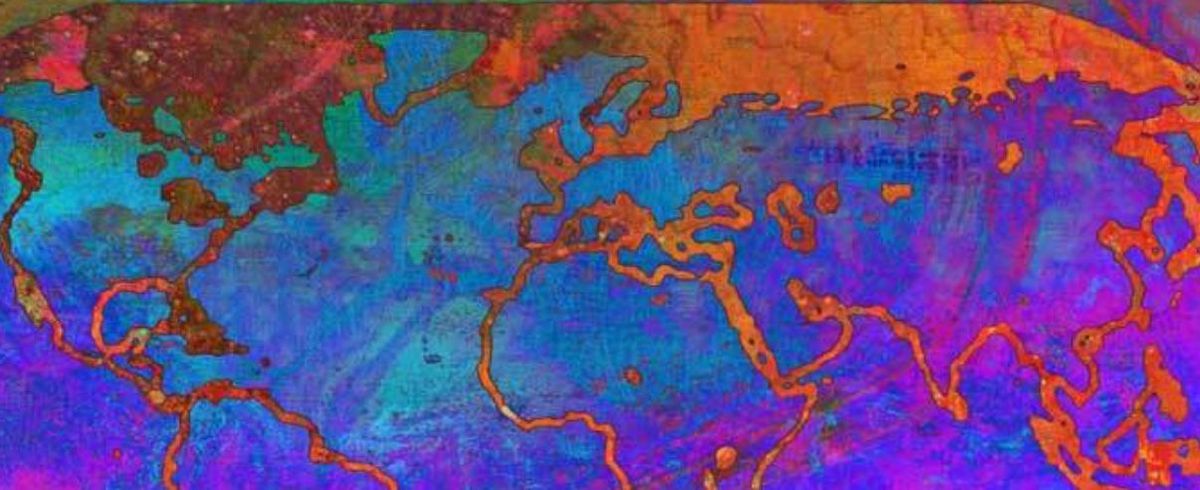
For building performance modelers, we can no longer rely on historical data for building energy modeling. We used historical typical meteorological year (TMY) files in the past today, we must opt for future weather files and prepare our buildings🏬 to be 🌡️ climate-proof. I strongly recommend using future weather 📂 files to better (more accurately) 🔣 design, equip and size our buildings and enable 🌡️ climate-proof architectural design.
📣 Read and share the 📄 tip: https://lnkd.in/eYcAZSjq
Thank you, Camille Gobin, Dru Crawley, Vahid Nik, Anaïs Machard, Agnese Salvati, Parag Rastogi, Xavier Fettweis, and Sébastien Doutreloup, for your inspiration.
Subscribe to my mailing list: www.shadyattia.org
Look at my ☑️ recent posts' activities. https://lnkd.in/eR-jiXtR
#buildingenergymodeling #climatechange #scenarios #futurefile #weatherdata #TMY #energysimulation #climateanalysis
According to the IPCC report (2021) [1], there is no longer any doubt that human activities have warmed the atmosphere, ocean, and lands. Climate change is becoming more widespread, faster, and more intense. Each of the last decades has been successively warmer than any decade since 1850.
For building performance modelers, we can no longer rely on historical data. We used historical typical meteorological year (TMY) files in the past [2]. A TMY file is a set of meteorological data with data values for every hour in a year for a given geographical location [3]. The data are selected from hourly data in a longer period (normally 20 years or more). 30 years is recommended as a standard averaging period for calculating quintile boundaries in climatological standard normals (World Meteorological Organization 2017). Today we must use future weather files with different scenarios and probabilities of occurrence.
According to Wahid Nik 2016 [4], there are four methods to create future climate scenarios:
1. Dynamic downscaling: (Physics-based model used to downscale global climate model results)
2. Analogue scenarios: (Find existing locations with comparable data to the predicted climate change scenario results)
3. Time series adjustment (morphing): (Shift and stretch the existing data to match the predicted monthly change)
4. Statistical models: (Stochastic model trained on observed data adjusts data based on altered frequency distributions of weather variables)
The most reliable approach is to follow the dynamic downscaling approach. It uses Global Climate Models (GSMs) or Earth System Models (ECMs) to be downscaled and coupled with statistical data on topography, land use, land cover, etc., to generate regional weather files. Dynamic downscaling can be done by climatologists or building engineers. However, the process is tedious and costly.
Solutions: For building energy modelers, I advise using dynamic downscale weather files developed nationally by recognized scientific peers or private service providers. If not, you can use commercial software like Meteonorm [5]. Meteonorm is a meteorological database/software that provides historical data and future weather data under climate change for 8325 cities. Future data for IPCC Scenarios from 10 global climate models based on CMIP5 are included (2020 - 2100).
Another approach could be using analog scenarios. The analog scenarios are very powerful and do not cost much. For example, I could replace the weather file in my city (Liege, Belgium) with Reims in France, 100 km to the South, with a 1 C degree equivalent increase. If you are in the Northern Hemisphere, you can apply the same rule of fetching a city 100 km south of your study city for 1 C degree increase scenarios or 2km south for a 2 C degree scenario. You reverse this approach by searching cities in the North if you live in the Southern Hemisphere. However, I need to consider the topographical similarity between both cities. Compared to a canyon or hilly city, a flat city will make a major climatic difference on the microscale level.
This brings me to the final advice regarding choosing future weather files. Under any circumstance, your future weather file will not represent the urban local climate. Most available weather files are extracted from airports located outside the city outskirts and do not represent the urban local climate. Therefore, your will need to estimate hourly air temperature and relative humidity in the urban canopy from a real weather station. If not, you will be left with the use of Approach 3. You can use commercial software like the Urban Weather Generator [6], which is an urban heat island effect modeling software. The output is a morphed weather file [epw] that captures the urban heat island effect and is compatible with many building performance simulation programs.
Finally, there is nothing like real weather data in accuracy and representation. So the most sustainable approach is installing a network of urban weather stations and updating the future weather file projections every 5-6 years in coordination with the IPCC reports prognosis.
The tips above are the tips that I share with my students. Don't hesitate to correct me or enlighten my followers by posting comments.
Good luck, fellow modelers; below, you can find some useful resources.
References
[1] IPCC Sixth Assessment Report: https://www.ipcc.ch/report/ar6/wg1/
[2] Barnaby & Crawley (2011). Weather data for building performance simulation in Hensen, & Lamberts, (eds.), Building performance simulation for design and operation
[3] https://app.ensims.com/epwmap.html
[4] Nik, V. (2016). Making energy simulation easier for future climate–Synthesizing typical and extreme weather data sets out of regional climate models (RCMs). Applied Energy, 177, 204–226.
[5] https://meteonorm.com/en/
[6] Bueno, B., Norford, L., Hidalgo, J., Pigeon, G. (2013). The urban weather generator, Journal of Building Performance Simulation. 6, 269-281.